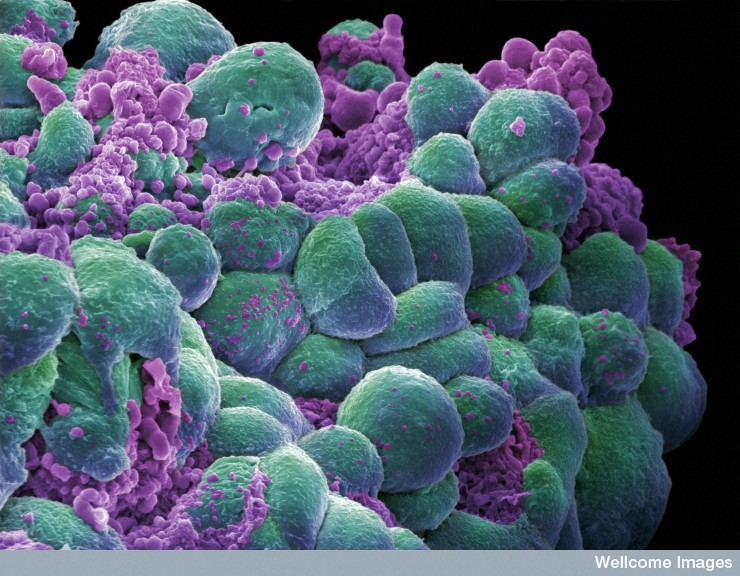
A cluster of breast cancer cells showing visual evidence of programmed cell death (apoptosis). Scanning electron micrograph false color
Ted Goldstein is not someone you would expect to find pursuing a doctorate in cancer research. After more than 25 years working in Silicon Valley, he left an executive-level position at Apple to return to grad school at his alma mater, UC Santa Cruz. UCSC’s Cancer Genomics Hub, led by David Haussler, serves as the number-crunching center for several large cancer studies. The key to its role as a cancer research powerhouse, says Goldstein, has to do with the kind of number-crunching they do: Bayesian updating.
Goldstein notes that when he graduated from UCSC in 1982, Bayes’ theorem, which is the basis of Bayesian statistics, got a cursory treatment in his undergraduate classes. In the intervening decades, it’s become a mainstay of the computer industry.
Only a handful of researchers, though, have applied it to cancer genomics (the study of the genetics of cancer cells). UCSC, along with Duke University, has one of the most mature programs using this approach, according to Goldstein, partly because of the computational expertise of the faculty in the Biomolecular Engineering department, especially Joshua Stuart, who runs the Systems Biology Group.
Bayesian updating, or Bayesian inference, rests on Bayes’ theorem. The theorem is simple, but the math behind it gets a little hairy. Bayesian updating is a way of incrementally updating your knowledge, and using the knowledge you gain at every step to inform your next experiment.
Goldstein likes to use the following analogy: If you have no experience with drinking alcohol and you go drinking every weekend, you are likely to get very drunk. But you’ll also be incrementally learning your tolerance for alcohol. Once you know four margaritas make you puke, you can use that information on your next night on the town.
The traditional approach to solving medical mysteries is to test one difference between comparison groups at a time — or at most a couple together. For example, you might look at the level of exercise between two groups of people who do or don’t have heart disease, or maybe exercise combined with diet. As an epidemiologist, that’s the way I’m used to thinking about disease. You survey a large group and see what risk factors the people who get sick have in common. This method works really well when there is one factor, or a few related factors, responsible for the illness. It’s how we figured out cigarettes cause lung cancer.
For something like breast cancer, where many possible genetic mutations can possibly lead to disease, that method hasn’t been as successful. Even the most common breast cancer
mutation, on the BRCA gene, only affects 15 percent of breast cancer patients. The rest are caused by a wide array of mutations, each of which might affect only one percent of all the cases.
Bayesian analysis lets you look at several different contributing factors at once. You also don’t need nearly as large sample groups for Bayesian inference as you do for old-fashioned epidemiological studies.
“Everyone’s cancer is not from the same pathogen. It’s unique,” says Goldstein. So the traditional approach “fails utterly. We just don’t have the numbers to bring to bear. We need another science. And that’s where Bayesian thinking comes in.”
We’re beginning to see the results of UCSC’s approach. UCSC researchers contributed to a large NIH study that reported on links between some types of breast and ovarian cancer and was reported in the journal Nature in September. Despite the hype and subsequent blowback that surrounded the study, it did report several new mutations that cause cancer, as well as the complex way the mutations work together.
The cure for cancer is still years in the future, but the work being done at UCSC’s Cancer Genomics Hub is a step in the right direction.